In compliance and litigation settings, we are routinely confronted by an opposing party alleging some form of inappropriate conduct. Disputes often arise involving overpayment allegations, audits of insurance claims, or purported false claims, and any of these allegations may invoke sampling and extrapolation as the basis for such claims. In those cases, an aptitude for evaluating and arguing statistical sampling analysis is valuable. This is the first of a two-part series addressing techniques for scrutinizing sampling analysis. arguing statistical sampling
Sampling requires a rigorous degree of technical expertise, thoughtful planning, and attentive execution to ensure the process is conducted objectively, and that it yields sound and reliable conclusions. Ensuring those requirements are met by opposing parties is equally important, therefore, this post highlights common areas for evaluating and scrutinizing opposing sampling and extrapolation analysis:
Sufficient Documentation and Personnel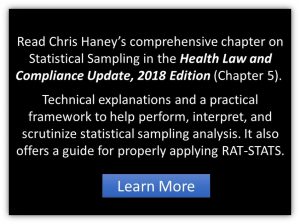
Sufficient documentation of an opposing analyst’s planning, design and execution must be provided to enable a reasonable assessment of their conclusions. Whether this data is produced voluntarily or through discovery, it should be complete and comprehensive. Specifically, it should include documentation and memorialization of any and all decision-making, calculations and findings for each and every step of the sampling framework. Without these details, it may be impossible to evaluate whether a particular analysis is valid or reliable, and subsequently, whether the analysis’ conclusions are meaningful. arguing statistical sampling
For this reason, a variety of industry guides require analysts to maintain relevant records of sampling analysis. For instance, the Centers for Medicare and Medicaid Services (“CMS”) requires, among other things, an explicit statement of how the population is defined, a specification of the units in the population, and that sufficient documentation be kept so the sampling frame can be re-created in the event of a challenge to the methodology. In fact, in audits of Medicare claims by recovery auditors, the Medicare Appeals Council (“MAC”) has rejected sampling analysis when sufficient documentation was not produced to the audited party.
Similarly, it can also be worthwhile to evaluate the credentials, education, training and experience of individuals responsible for performing analysis. Here again, standards such as the AICPA’s Generally Accepted Auditing Standards mandate sufficient technical expertise, while CMS goes further to specify the level of statistical expertise involved.
Generally Accepted Standards
Determining what procedures or guidelines were purportedly followed by an analyst, and whether or not they properly conformed to those guidelines, can provide a meaningful assessment of a particular analysis’ quality. Although statistical sampling has been upheld in courts as an accepted method of estimation for decades, courts have not adopted specific guidelines for sampling methodologies. In fact, MACs and federal courts have both held that there is no formal recognition of “generally accepted statistical principles and procedures.”[1] However, this should not be interpreted to mean that no principles and procedures exist. Instead, these rulings only highlight that no single principle or procedure governs the validity or usefulness of all sampling procedures.
For instance, recovery contractors conducting audits of Medicare providers are required to abide by the Medicare Program Integrity Manual (“MPIM”), which includes guidelines on data analysis, statistical sampling, extrapolation, and estimation of overpayments. Similarly, a provider performing sampling analysis for the purposes of self-disclosure to OIG is required to conform to OIG’s Provider Self-Disclosure Protocol (“SDP”), which specifies certain sample design requirements. These guidelines do not control in every instance, however they are useful standards for certain circumstances. In addition to these guidelines, contractual agreements between parties may also stipulate certain agreed-upon principles or procedures with regard to sampling analysis.
Sample Size
A common strategy when scrutinizing sampling analysis involves addressing the size of an opposing sample. Since larger sample sizes generally increase precision, many argue a sample size is ‘too small’ when they believe the conclusions to be invalid. Such arguments are rarely successful on their own. Recall from our earlier posts about sample size that even a seemingly small sample can generate meaningful and valid conclusions when properly designed and executed. Once again, the obstacle is that no formal standard exists in which a minimum sample size is stipulated. However, court rulings regarding minimum sample size should not be interpreted to mean that no standard exists. For example, OIG has published its own guidance regarding sample size thresholds in its SDP. Among other things, the SDP requires the submission of a detailed sampling plan with a sample size of at least 100 claims. See our post about Determining Sample Size for additional considerations.
Randomness of the Sample
Another common area for scrutiny involves how the sample was selected. Recall that the Forensus’ Sampling Framework describes statistical sampling, which requires the selection and analysis of truly random samples. In other words, haphazard or convenience samples should not be used for the purposes of calculating an objective confidence interval. Scrutiny should be focused on confirming whether a sample was actually designed and selected randomly (i.e. Steps 3 & 5 of Forensus’ Framework).
While the MAC and federal courts have widely held that software programs such as RAT-STATS are a reliable means of selecting a sample, these programs are only as effective as their operator. Appellants should scrutinize the opposing analyst’s work plan to ensure RAT-STATS was used as intended and that the resulting outputs were properly employed.
Errors in the selection of a sample or intentionally including specific claims in an otherwise random selection (i.e. cherry-picking) may result in biased and invalid conclusions. For instance, in Sanders, a case involving RAC overpayment demands, the MAC found that “… either the samples themselves were not drawn correctly or the claims were not correctly assigned to the correct stratum in every case consistent with the probability sample design.”[2] Due in part to this finding of improper sampling, the extrapolation was excluded and overpayments were limited to the actual claims sampled. This case highlights that simply using RAT-STATS does not protect against scrutiny, much like using a calculator may not prevent a calculation error.
… Click Here to see Part Two of our series discussing Arguing Statistical Sampling and Extrapolation
Arguing Statistical Sampling Refuting Statistical Sampling
Invalid Statistical Sampling
©Forensus Group, LLC | 2017
[1] Michael King, M.D. and Kinston Medical Specialists, P.A. (Appellant) (Beneficiaries) Cigna Government Services (Contractor), Claim for Part B Benefits, 2011 WL 6960267 (May 10, 2011); see also Pruchniewski v. Leavitt, 2006 WL 2331071, 8:04-CV-2200-T-23TBM (M.D. Fla).
[2] Sanders, 2011 WL 6960281, (H.H.S. May 12, 2011).